New Tool Can Better Select Most Promising Gene Candidates For Research, Study Suggests
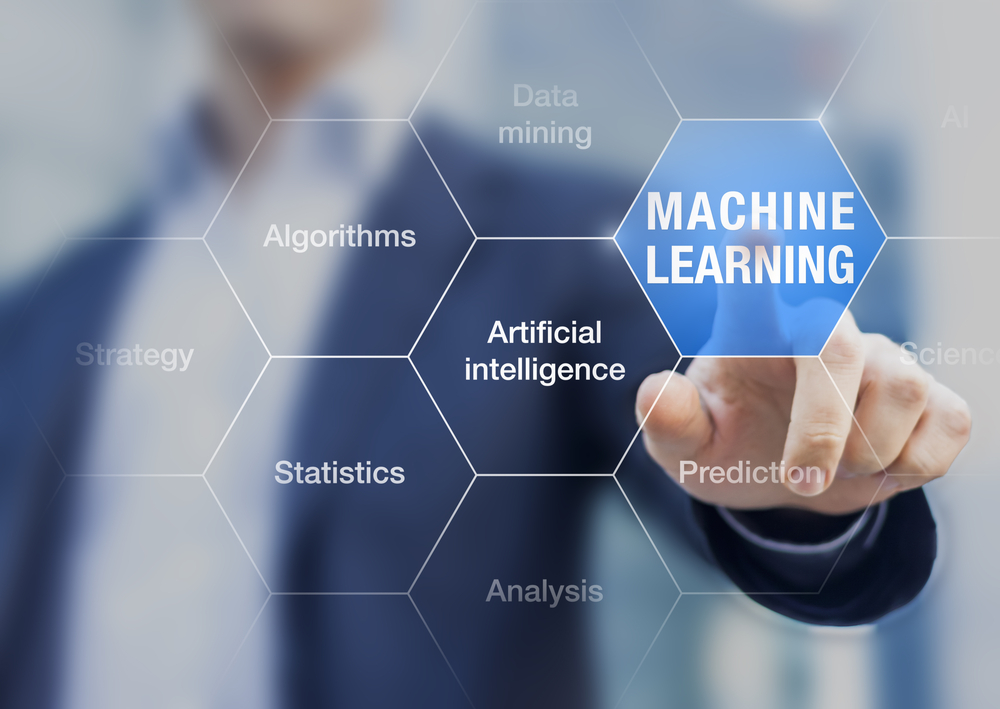
Scientists have created a new tool that allows them to look in an unbiased manner for genes that may be involved in several traits, such as a person’s height or risk of developing obesity, a study suggests.
The study, “Benchmarker: An Unbiased, Association-Data-Driven Strategy to Evaluate Gene Prioritization Algorithms,” was published in The American Journal of Human Genetics.
Genome-wide association studies (GWAS) are based on a method that scans the genome (all of the genes present in our DNA) looking for specific types of genetic alterations found more frequently in people with a particular disease.
Usually, these studies come up with thousands of genes and genetic variants that may potentially be interesting to investigate further.
Scientists normally select the genes and variants for further research by using mathematical algorithms that have been designed to prioritize specific sets of genes or variants considered more likely to be involved in the disease or trait they are investigating.
The problem with using such algorithms is that genes that are deemed “less likely” to be involved in a particular disease or trait will not show up in the final selection of gene candidates.
As a result, scientists tend to look at those gene candidates that have a higher chance of being involved in a particular disorder, and eventually disregarding the contribution of others that are unknown and that may advance their field of research.
For this reason, it’s important to select the best possible algorithm that sorts genes in an unbiased way for each particular disorder.
“We have different prioritization algorithms, but we don’t actually know how to decide which one is best,” Rebecca Fine, a PhD student at Harvard Medical School and lead author of the study, said in a news release. “We didn’t want to have to rely on a previous ‘gold standard’ or bring in anything other than the original GWAS data.”
In this study, a group of researchers from Harvard Medical School and their collaborators described the use of a new tool called Benchmarker, which allows them to compare the performance of several prioritization algorithms so that they are able to determine the best one to use in each particular case.
Benchmarker works by using data from the GWAS study as its own internal control. It leaves out one chromosome, which will be used as a control, and then allows the prioritization algorithms to use information from the remaining 21 chromosomes (all except the X and Y chromosomes) to figure out which genes on the withheld chromosome may be the most promising candidates to study for a particular disease or trait.
The process is then repeated for all the other chromosomes, while the top gene candidates selected by the prioritization algorithm are pooled. The performance of each prioritization algorithm is calculated by comparing the group of pooled genes to the original findings of the GWAS study.
In the study, the researchers put Benchmarker to the test using GWAS data to compare the performance of three different prioritization algorithms — DEPICT, MAGMA, and NetWAS — on 20 different traits, including height, body mass index, skin tone, cholesterol levels, triglycerides levels, and blood pressure. They also tested combinations of different methods.
By doing so, they found that in general DEPICT and MAGMA outperformed NetWAS at selecting the best gene candidates for each trait. However, they also discovered that combining different methods often allowed them to achieve the best results.
Finally, they also found evidence that some prioritization algorithms work better to look for gene candidates associated with specific traits.
“We believe that the overall cross-validation approach described and implemented here provides a better ‘gold standard’ for benchmarking existing and future methods for gene and variant prioritization. Finally, Benchmarker can be used to determine the best algorithm and dataset for any particular trait of interest,” the scientists wrote.